Research master student Mingxuan Song presented poster at NESG 2024
Research master student Mingxuan Song was invited to present a poster at The Netherlands Econometric Study Group (NESG) 2024 conference.
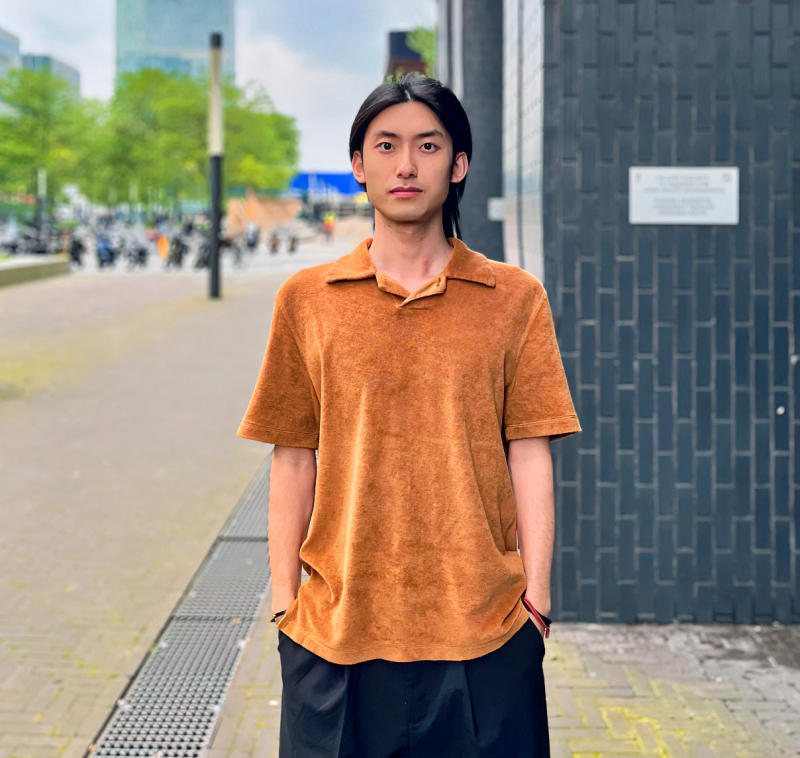
Mingxuan presented the paper 'Bootstrap inference for linear time-varying coefficient models in locally stationary time series.'
Also PhD students Floris Holstege (University of Amsterdam) and Bernhard van der Sluis (Erasmus University Rotterdam) present a poster during NESG 2024. Floris on 'Removing Spurious Concepts from Neural Network Representations via Joint Subspace Estimation' and Bernhard on 'Markov regime-switching panel models with grouped heterogeneity'
The conference took place at Maastricht University School of Business and Economics on May 24-25. Keynote speaker of this year's conference was Michael Jansson (UC Berkeley, United States).
Article citation
Yicong Lin, Mingxuan Song, Bernhard van der Sluis, Bootstrap inference for linear time-varying coefficient models in locally stationary time series.
Abstract
Time-varying coefficient models can capture evolving relationships. However, constructing asymptotic confidence bands for coefficient curves in these models is challenging due to slow convergence rates and the presence of various nuisance parameters. A residual-based sieve bootstrap method has recently been proposed to address these issues. While it successfully produces confidence bands with accurate empirical coverage, its applicability is restricted to strictly stationary processes. We introduce a new bootstrap scheme, the local blockwise wild bootstrap (LBWB), that allows for locally stationary processes. The LBWB can replicate the distribution of the parameter estimates while automatically accounting for nuisance parameters. An extensive simulation study reveals the superior performance of the LBWB compared to various benchmark approaches. It also shows the potential applicability of the LBWB in broader scenarios, including time-varying cointegrating models. We then examine herding effects in the Chinese renewable energy market using the proposed methods. Our findings strongly support the presence of herding behaviors before 2016, aligning with earlier studies. However, contrary to previous research, we find no significant evidence of herding between around 2018 and 2021.
Photo: Mingxuan Song