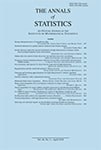
Can, S., Einmahl, J., Khmaladze, E. and Laeven, R. (2015). Asymptotically distribution-free goodness-of-fit testing for tail copulas The Annals of Statistics, 43(2):878--902.
-
Affiliated author
-
Publication year2015
-
JournalThe Annals of Statistics
Let (X1,Y1),…,(Xn,Yn) be an i.i.d. sample from a bivariate distribution function that lies in the max-domain of attraction of an extreme value distribution. The asymptotic joint distribution of the standardized component-wise maxima ⋁ni=1Xi and ⋁ni=1Yi is then characterized by the marginal extreme value indices and the tail copula R. We propose a procedure for constructing asymptotically distribution-free goodness-of-fit tests for the tail copula R. The procedure is based on a transformation of a suitable empirical process derived from a semi-parametric estimator of R. The transformed empirical process converges weakly to a standard Wiener process, paving the way for a multitude of asymptotically distribution-free goodness-of-fit tests. We also extend our results to the m-variate (m>2) case. In a simulation study we show that the limit theorems provide good approximations for finite samples and that tests based on the transformed empirical process have high power.