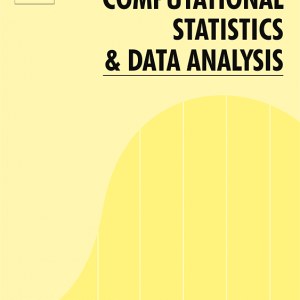
Koopman, S., Hindrayanto, A. and Ooms, M. (2010). Exact maximum likelihood estimation for non-stationary periodic time series models Computational Statistics and Data Analysis, 54:2641--2654.
-
Affiliated authorsIrma Hindrayanto, Siem Jan Koopman
-
Publication year2010
-
JournalComputational Statistics and Data Analysis
Time series models with parameter values that depend on the seasonal index are commonly referred to as periodic models. Periodic formulations for two classes of time series models are considered: seasonal autoregressive integrated moving average and unobserved components models. Convenient state space representations of the periodic models are proposed to facilitate model identification, specification and exact maximum likelihood estimation of the periodic parameters. These formulations do not require a priori (seasonal) differencing of the time series. The time-varying state space representation is an attractive alternative to the time-invariant vector representation of periodic models which typically leads to a high dimensional state vector in monthly periodic time series models. A key development is our method for computing the variancecovariance matrix of the initial set of observations which is required for exact maximum likelihood estimation. The two classes of periodic models are illustrated for a monthly postwar US unemployment time series. {\textcopyright} 2010 Elsevier B.V. All rights reserved.