Machine learning and individuals in the digital society
In his inaugural address Learning with a purpose: the balancing acts of machine learning and individuals in the digital society, Professor Gui Liberali (Erasmus University Rotterdam and Erasmus Medical Center) shows how advances in machine learning and reinforcement learning can alleviate challenging balancing acts, faced by firms and consumers.
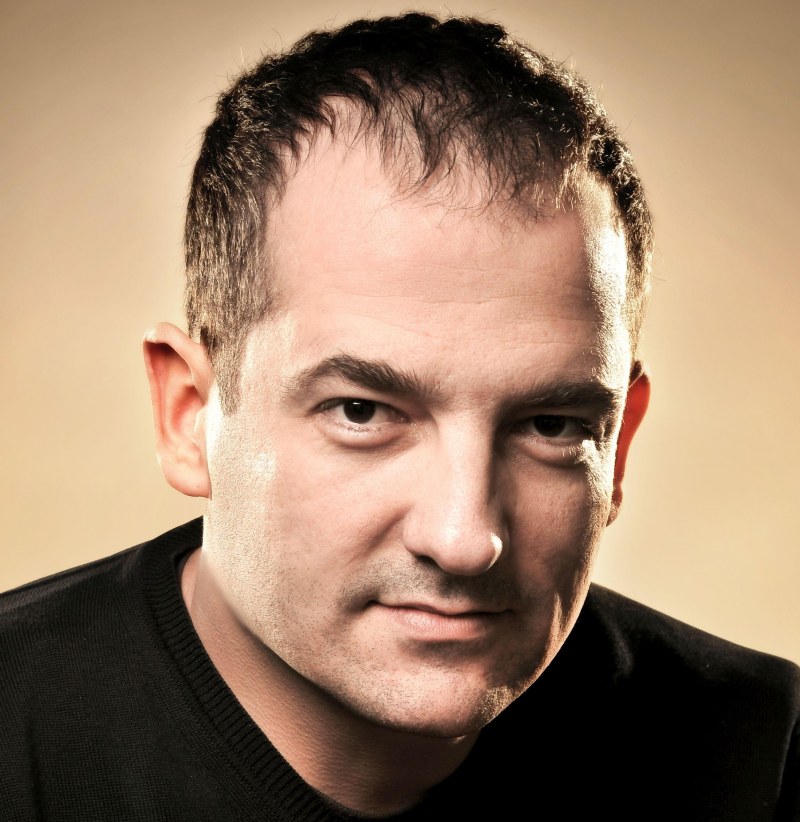
Liberali presented his inaugural address on Friday May 25, 2018.
About the inaugural address
The Internet transformed some of the most basic processes in our society, such as trade, payment, and communication. We now have more access to products, services, and opinions than we ever had, but at the same time, our behaviour is tracked more closely than ever. For example, large online retailers offer hundreds of thousands of products, and can readily observe in great detail how each consumer interacts with any them. They can also rapidly deploy individual-level, in-vivo, randomized online experiments at population scale to test concepts, insights and communication approaches which can lead to better services and products. However, there are often billions of possibilities, such as product-consumer combinations for product recommendations. The scale and complexity of these experiments create amazing challenges.
Thus, firms face balancing acts. For example, they need to constantly choose between profiting from what they already know about consumers (such as the genres of movies already watched) and learning more about the same consumers (such as by recommending a movie of an untested genre). Consumers are also facing their own balancing acts. In the digital society, we inevitably leave digital footprints but we have some discretion in terms of how much information we want to keep private. Typically, a consumer that is more open to sharing her preferences is also exposed to higher risks, but at the same time she can get better access to products and services she needs.
In this talk, Professor Liberali shows how advances in machine learning and reinforcement learning can alleviate these challenging balancing acts. After providing some background information, he briefly describes how these methods are helping firms and consumers, illustrating with his own work. Then he indicated key exciting areas for future research. He concluded this address by illustrating the implications for marketing science and prescriptive analytics more generally.