Gui Liberali published in Marketing Science
Business Data Science faculty member Gui Liberali published a paper entitled Morphing for Consumer Dynamics: Bandits Meet Hidden Markov Models in the journal Marketing Science. Liberali wrote his paper in collaboration with Alina Ferecatu from the Rotterdam School of Management (RSM).
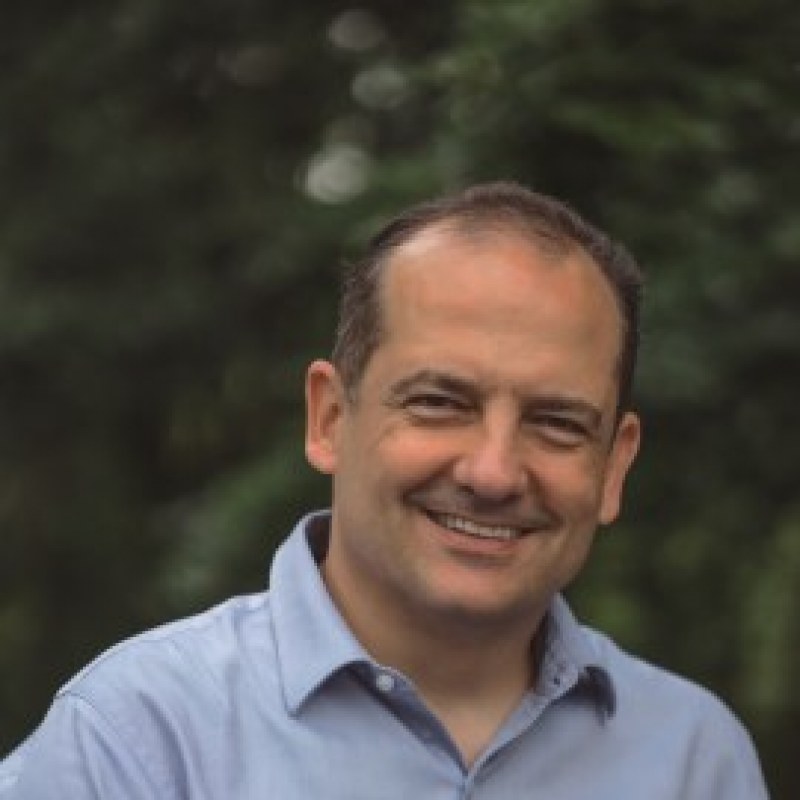
Paper abstract: Websites are created to help visitors take an action, such as making a purchase or a donation. As visitors browse various web pages, they may take rapid steps toward the action or may bounce away. Websites that can adapt to match such consumer dynamics perform better. However, assessing visitors’ changing distance to the action, at each click, and adapting to it in real time is challenging because of the sheer number of design elements that are found in websites, that combine exponentially. We solve this problem by matching latent states to web page designs, combining recent advances in multiarmed bandit (MAB), website morphing, and hidden Markov models (HMM) literature. We develop a novel dynamic program to explicitly model the trade-off firms face between nudging a visitor to later states along the funnel, and maximizing immediate reward given current estimates of purchase probabilities. We use an HMM to assess visitors’ states in real time, and couple it with an MAB model to learn the effectiveness of each design × state combination. We provide a proof of concept in two applications. First, we conduct a field study on the Master of Business Administration website of a major university. Second, we implement our algorithm on a cloud server and test it on an experimental online store.
Click here to read the full paper.
Summer School with Gui Liberali on Reinforcement Learning, August 22-26, 2022
Sharpen your machinelearning skillls (more specifically, in multi-armed bandits and reinforcementlearning) this summer at Campus Woudestein, Erasmus University Rotterdam.
Gui will be running a one-week hands-on Summer course on the fundamentals and applications of multi-armed bandits. Open to and designed for students and professionals working in areas such as, but not limited to: ABtesting, online advertising, pricing, website optimization, targeting, coupons, personalization, and any sequential decision-making problems or learning algorithms.