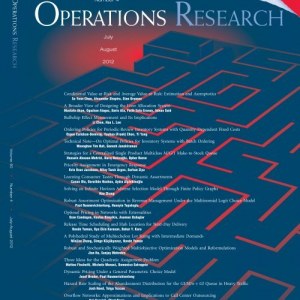
Peng, Y., Fu, MichaelC., Hu, J.Q. and Heidergott, B. (2018). A new unbiased stochastic derivative estimator for discontinuous sample performances with structural parameters Operations Research, 66(2):487--499.
-
Affiliated author
-
Publication year2018
-
JournalOperations Research
In this paper, we propose a new unbiased stochastic derivative estimator in a framework that can handle discontinuous sample performances with structural parameters. This work extends the three most popular unbiased stochastic derivative estimators: (1) infinitesimal perturbation analysis (IPA), (2) the likelihood ratio (LR) method, and (3) the weak derivative method, to a setting where they did not previously apply. Examples in probability constraints, control charts, and financial derivatives demonstrate the broad applicability of the proposed framework. The new estimator preserves the singlerun efficiency of the classic IPA-LR estimators in applications, which is substantiated by numerical experiments.