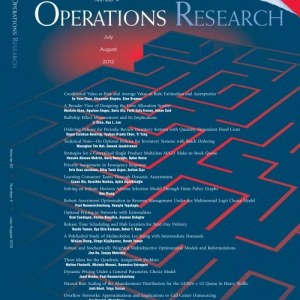
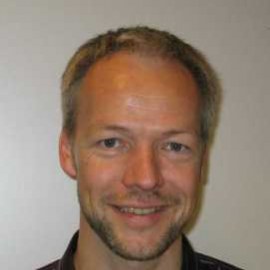
Bernd Heidergott
Biography
Bernd Heidergott is professor of Stochastic Optimization at the department of Econometrics and Operations Research of the Vrije Universiteit Amsterdam. He is working at the VU since 2002. Before that he worked at the TU Eindhoven, at EURANDOM, at DIAM, and at the Erasmus University Rotterdam. He obtained his PhD in 1996 at the University of Hamburg, Germany.
Currently, he is Program Director Econometrics and Operations Research and Board Member of Amsterdam Business Research Institute.
Key publications
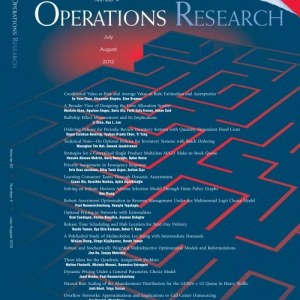
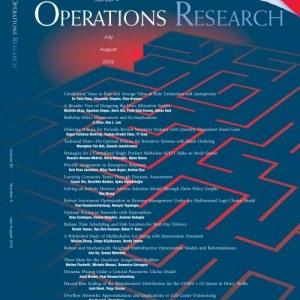
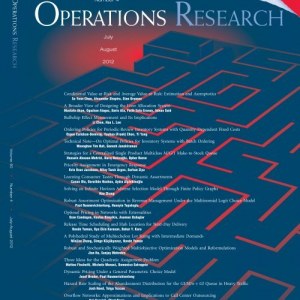
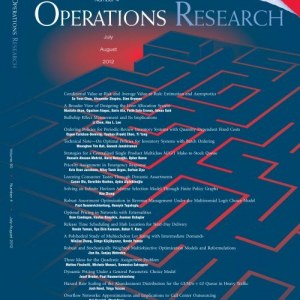
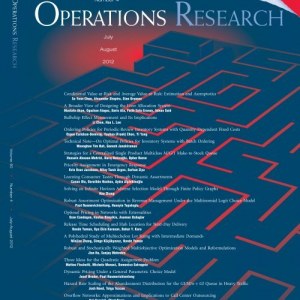
Publications
Volk-Makarewicz, W., Borovkova, S. and Heidergott, B. (2022). Assessing the impact of jumps in an option pricing model: A gradient estimation approach European Journal of Operational Research, 298(2):740--751.
Peng, Y., Xiao, L., Heidergott, B., Hong, L.Jeff and Lam, H. (2022). A New Likelihood Ratio Method for Training Artificial Neural Networks INFORMS Journal on Computing, 34(1):638--655.
Peng, Y., Fu, MichaelC., Heidergott, B. and Lam, H. (2020). Maximum likelihood estimation by monte carlo simulation: Toward data-driven stochastic modeling Operations Research, 68(6):1896--1912.
Berkhout, J. and Heidergott, BerndF. (2019). Analysis of Markov influence graphs Operations Research, 67(3):892--904.
Peng, Y., Fu, MichaelC., Hu, J.Q. and Heidergott, B. (2018). A new unbiased stochastic derivative estimator for discontinuous sample performances with structural parameters Operations Research, 66(2):487--499.
Heidergott, B. and Volk-Makarewicz, W. (2016). A measure-valued differentiation approach to sensitivities of quantiles Mathematics of Operations Research, 41(1):293--317.
Goverde, R., Heidergott, B. and Merlet, G. (2011). A coupling approach to estimating the Lyapunov exponent of stochastic max-plus linear systems European Journal of Operational Research, 210(2):249--257.
Heidergott, B., Hordijk, A. and Leder, N. (2010). An approximation approach for the deviation matrix of continuous-time Markov processes with application to Markov decision theory Operations Research, 58:918--932.
Heidergott, B., Hordijk, A. and Leder, N. (2010). Series expansions for continuous-time Markov chains Operations Research, 58:756--767.
Heidergott, B. and Leahu, H. (2010). Weak differentiability of product measures Mathematics of Operations Research, 35:27--51.
Heidergott, B. and Farenhorst - Yuan, T. (2010). Gradient estimation for multicomponent maintenance systems with age-replacement policy Operations Research, 58:706--718.
Heidergott, B., Vazquez-Abad, F. and Volk Makarewicz, W.M. (2008). Sensitivity Estimation for Gaussian Systems European Journal of Operational Research, 187:193--207.
Heidergott, B., Hordijk, A. and Weisshaupt, H. (2006). Measure-valued differentiation for stationary Markov chains Mathematics of Operations Research, 31:154--172.
Heidergott, B., de Kort, A. and Ayhan, H. (2003). A probabilistic approach for determining railway infrastructure capacity European Journal of Operational Research, 148:644--661.
Heidergott, B. (2001). A differential calculus for random matrices with applications to (max,+)-linear stochastic systems Mathematics of Operations Research, 26(4):679--699.
Heidergott, B. (1999). Optimization of a single-component maintenance system: a smoothed perturbation analysis approach European Journal of Operational Research, :181--190.