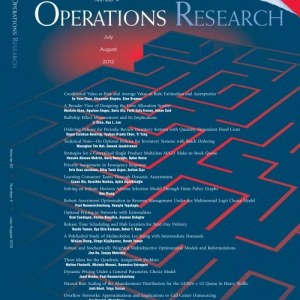
Peng, Y., Fu, MichaelC., Heidergott, B. and Lam, H. (2020). Maximum likelihood estimation by monte carlo simulation: Toward data-driven stochastic modeling Operations Research, 68(6):1896--1912.
-
Affiliated author
-
Publication year2020
-
JournalOperations Research
We propose a gradient-based simulated maximum likelihood estimation to estimate unknown parameters in a stochastic model without assuming that the likelihood function of the observations is available in closed form. A key element is to develop Monte Carlo-based estimators for the density and its derivatives for the output process, using only knowledge about the dynamics of the model. We present the theory of these estimators and demonstrate how our approach can handle various types of model structures. We also support our findings and illustrate the merits of our approach with numerical results.