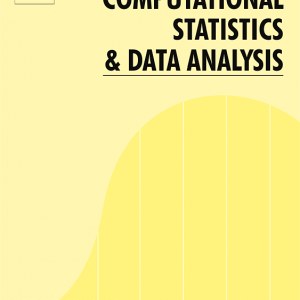
Schaumburg, J. (2012). Predicting extreme Value at Risk: Nonparametric quantile regression with refinements from extreme value theory Computational Statistics and Data Analysis, 56(12):4081--4096.
-
Affiliated author
-
Publication year2012
-
JournalComputational Statistics and Data Analysis
A framework is introduced allowing us to apply nonparametric quantile regression to Value at Risk (VaR) prediction at any probability level of interest. A monotonized double kernel local linear estimator is used to estimate moderate (1%) conditional quantiles of index return distributions. For extreme (0.1%) quantiles, nonparametric quantile regression is combined with extreme value theory. The abilities of the proposed estimators to capture market risk are investigated in a VaR prediction study with empirical and simulated data. Possibly due to its flexibility, the out-of-sample forecasting performance of the new model turns out to be superior to competing models. {\textcopyright} 2012 Elsevier B.V. All rights reserved.